BeamLearning - Source localization using Deep Learning
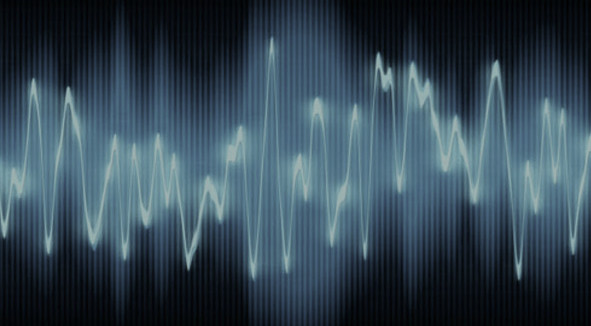
Smart microphone arrays - Source localization using Deep Learning
Supervised student : Hadrien Pujol
Supervision : Éric Bavu , Alexandre Garcia
Project duration : 2018 - 2020
Funding : Ministerial grant
Abstract : Over the last three decades, the technological growth of microphone arrays has accompanied the development of numerous acoustic signal processing algorithms for acoustic source localization. The field has now reached such a maturity that this type of device is now used in a large number of industrial and domestic systems, including those accessible to the general public. Much of the current research in acoustics makes use of microphonic antennas of various topologies, to perform tasks as varied as structural inspection, source localization, denoising, source separation, de-reverberation or spatialized capture.
Acoustic imaging is the core application of the work done for more than 30 years by the LMSSC acoustics researchers. The algorithms developed by the scientific community reach today a great maturity. Most of them are based on a physical model of the propagation environment, or on statistical hypotheses about the sources or the sound signal they emit. The major problem faced by the scientific community concerns the robustness and accuracy of these methods as soon as the measurement environment is poorly known, and the sources or signals deviate from the assumptions made for the resolution of the inverse problems. To overcome this problem, we propose in this project to develop the use of emerging techniques in artificial intelligence to address the problem of localization of unknown sound sources, in a complex environment also unknown.
Figure : Deep Learning localization approach: the input data are the audio signals (multichannel) captured on the microphone antenna. The objective of the network is to build a representation of the data capable of deducing the position of the source that emitted the sound wave.
The proposed approach allows minimizing the assumptions on the relevant information to be extracted from the data measured by the sensors of the microphonic antenna, but also on the propagation model and the measurement environment. These tasks will be entrusted to a deep neural network (Deep Learning), which will make it possible to propose massive data processing and localization algorithms that evolve with the measurement environment, the sources present, but also the layout or the number of sensors composing the microphone antenna. The main objective of the approach is thus to make the localization of sources much more robust and less sensitive to the underlying model, since the treatments will be learned and built by the neural network to be sufficiently adaptable to the measurement environment or sources.
First results (not updated since 2019 - see latest publications for more up to date results)
Since the beginning of Hadrien Pujol’s thesis, we have already obtained encouraging results, since the neural network developed allows to localize a source in free field with an angular accuracy of about $1^{o}$, both using digitally simulated data, but also experimental data acquired on a compact 7-sensor microphone antenna.
Figure : Top: compact antenna of 7 MEMS microphones used for experimental validation of artificial intelligence - Bottom: 3D spatializer (Spherbedev) used to synthesize signals from selected 3D positions. For the experimental validation, the microphone antenna is placed in the center of the spatialization sphere, at the level of the physically valid reconstruction zone.
We have also shown that this approach allows us to implicitly calibrate the antenna microphones, thus providing superior localization capabilities to commonly used algorithms, in the case of poorly calibrated antennas (which was the case during the experimental validation, where no antenna calibration was performed).
Figure : Convergence of neural network learning for source localization using Deep Learning: numerical results and experimental validation.
Figure : Localization error rate by several commonly used methods for source localization, compared to the developed Deep Learning approach, in case the antennas are not calibrated.
In the continuation of the project, we will also treat the problem in a reverberant environment, by using a database of impulse responses of rooms, which will make it possible to carry out the training by exploiting (or by freeing itself) from the characteristics of the room in which the source emits the sound.
Publications and communications related to the project
- Hadrien Pujol, Eric Bavu, Alexandre Garcia, Localisation 3D de sources acoustiques par Deep Learning et entrainement de réseaux par spatialisation ambisonique d’ordres élevés, 16ème Congrès de la Société Française d’Acoustique 2022 Marseille, Apr 2022
- Hadrien Pujol, Eric Bavu, Alexandre Garcia, BeamLearning: an end-to-end Deep Learning approach for the angular localization of sound sources using raw multichannel acoustic pressure data , The Journal of the Acoustical Society of America, Vol 149-6, 4248–4263, 2021. doi
- Hadrien Pujol, Eric Bavu, Alexandre Garcia, 3D Sound source localization in reverberant rooms using Deep Learning and microphone arrays : Simulation and experiment , Forum Acusticum 2020 Lyon , Apr 2020
- Hadrien Pujol, Eric Bavu, Alexandre Garcia. Source localization in reverberant rooms using Deep Learning and microphone arrays , 23rd International Congress on Acoustics (ICA 2019 Aachen), Sep 2019, Aachen, Germany.
- Hadrien Pujol, Eric Bavu, Alexandre Garcia. Constitution d’une base de données physiquement valide pour les approches de localisation de sources par Deep Learning sur antennes microphoniques intelligentes , 14ème Congrès Français d’Acoustique, Le Havre, Apr 2018.
- Eric Bavu, Hadrien Pujol, Alexandre Garcia, Antennes non calibrées, suivi métrologique et problèmes inverses : une approche par Deep Learning , 14ème Congrès Français d’Acoustique, Le Havre, Apr 2018.
- Hadrien Pujol, Eric Bavu, Alexandre Garcia. Antennes microphoniques intelligentes : Localisation de sources par Deep Learning , 14ème Congrès Français d’Acoustique, Le Havre, Apr 2018.